A Complete Guide to the FDA’s AI/ML Guidance for Medical Devices
Table of Contents
A Complete Guide to the FDA’s AI/ML Guidance for Medical Devices
Artificial intelligence (AI) and machine learning (ML) technologies are rapidly transforming healthcare by extracting critical insights from the enormous amounts of data generated in the healthcare sector daily. These technologies are driving innovation in medical devices, enhancing their ability to support healthcare providers and improve patient outcomes. The ever-evolving nature of AI development, deployment, and maintenance demands stringent change management throughout the entire product lifecycle. The FDA has been proactive in pushing the industry towards safe AI/ML practices.
In this article, we will cover the most significant publications the FDA has released on AI/ML-enabled medical devices and provide a summary of each publication.
Understanding Artificial Intelligence and Machine Learning in Medical Devices
AI refers to computational systems capable of making predictions, recommendations, or decisions that influence real or virtual environments based on a set of human-defined objectives. These systems utilize a combination of machine- and human-derived inputs to perceive environments, abstract these perceptions into models through automated analysis, and use these models to generate options for action or information.
Machine learning, a subset of AI, comprises techniques used to train AI algorithms, enabling them to improve their performance on specific tasks through data exposure.
Examples of AI and ML in healthcare include:
- Diagnostic Imaging: AI-powered imaging systems that provide diagnostic insights for conditions like skin cancer (companies like DeepHealth/RadNet).
- Predictive Analytics: Smart devices that estimate the likelihood of cardiovascular events, such as heart attacks.
Transforming Medical Devices with AI and ML
AI and ML technologies are revolutionizing healthcare by providing new insights from daily healthcare data. Medical device manufacturers are leveraging these technologies to innovate and improve their products, thereby enhancing patient care. A significant advantage of AI/ML-based software is its capacity to learn from real-world usage, continually refining and enhancing its performance over time.
FDA's Regulatory Approach to AI/ML-Enabled Medical Devices
The FDA evaluates medical devices through various premarket pathways, including premarket clearance (510(k)), De Novo classification, and premarket approval. The agency also reviews modifications to existing devices, particularly when these involve software, to ensure that changes do not increase the risk to patients.
Traditional FDA regulatory frameworks were not initially designed for the adaptive nature of AI/ML technologies. As a result, the FDA has identified that many modifications to AI/ML-driven devices may require premarket review.
Key FDA AI Guidance Publications
- April 2019: “Proposed Regulatory Framework for Modifications to AI/ML-Based Software as a Medical Device (SaMD)”
- January 2021: “AI/ML SaMD Action Plan”
- October 2021: “Good Machine Learning Practice for Medical Device Development: Guiding Principles”
- April 2023: “Predetermined Change Control Plans for Machine Learning-Enabled Medical Devices: Guiding Principles”
- June 2024: “Transparency for Machine Learning-Enabled Medical Devices”
- August 2024: “Predetermined Change Control Plans for Medical Devices: Draft Guidance for Industry and FDA Staff”
- December 2024: "Marketing Submission Recommendations for a Predetermined Change Control Plan for Artificial Intelligence-Enabled Device Software Functions"
- January 2025: “Artificial Intelligence-Enabled Device Software Functions: Lifecycle Management and Marketing Submission Recommendations”
Below, we will briefly cover the main topics and functions of FDA’s major AI/ML guidances.
These documents collectively represent the FDA's commitment to fostering innovation while ensuring the safety and efficacy of AI/ML-enabled medical devices.
Summary of FDA’s Proposed Regulatory Framework for Modifications to AI/ML-Based Software as a Medical Device (SaMD)
AI-enabled medical devices utilize either adaptive or locked algorithms, each with distinct characteristics. Locked algorithms remain unchanged after deployment, while adaptive algorithms evolve based on new data. Although adaptive algorithms offer ongoing learning and improvement, they also require rigorous change management to maintain safety and effectiveness.
A Total Product Lifecycle (TPLC) approach is essential for managing AI/ML-enabled medical devices. This method integrates premarket evaluation with continuous post-market monitoring, ensuring devices remain safe and effective throughout their use.
Real-world performance monitoring is crucial for AI/ML-enabled devices as they rely on real-world data to refine their functions. Developers should establish robust systems to track device performance in various settings to ensure safety and effectiveness.
Transparency and explainability are vital for the trust and widespread adoption of AI/ML technologies in healthcare. Developers must ensure their models are transparent, with clear documentation of the algorithm's design, data used, and decision-making logic.
Good Machine Learning Practices (GMLP) are critical for the consistent development of AI/ML technologies. GMLP ensures the quality of data, robustness of algorithms, and systematic handling of updates.
Lastly, regulatory flexibility is key in the rapidly evolving AI/ML landscape. It allows developers to update their systems post-approval without needing full premarket submissions, provided changes are pre-approved under a predetermined change control plan. This approach supports innovation while maintaining safety and efficacy standards.
Summary of FDA’s AI/ML SaMD Action Plan
The AI/ML-Based Software as a Medical Device Action Plan sets forth five key initiatives that the FDA plans to pursue. These initiatives include:
- Further refining the regulatory framework, particularly by issuing draft guidance on predetermined change control plans to manage software updates.
- Promoting the development and adoption of good machine learning practices to ensure that AI/ML algorithms are rigorously evaluated and continuously improved.
- Encouraging a patient-focused approach by enhancing the transparency of devices to end users.
- Creating and refining methodologies for the assessment and enhancement of machine learning algorithms.
- Advancing initiatives for real-world performance monitoring to track how these devices function in practical, everyday scenarios.
This approach ensures that AI/ML technologies in medical devices are both innovative and aligned with safety and efficacy standards.
Summary of Good Machine Learning Practice for Medical Device Development: Guiding Principles
The U.S. Food and Drug Administration (FDA), in collaboration with Health Canada and the UK's Medicines and Healthcare products Regulatory Agency (MHRA), has established 10 guiding principles to shape the development of Good Machine Learning Practice (GMLP). These principles aim to ensure that AI and machine learning (ML) technologies used in medical devices are safe, effective, and of high quality.
Here are the 10 guiding principles for Good Machine Learning Practice (GMLP) identified by the FDA, Health Canada, and the UK's MHRA, along with brief descriptions of each:
- Multi-Disciplinary Expertise Throughout the Product Lifecycle: Involves incorporating diverse expertise to understand how AI/ML models will integrate into clinical workflows, ensuring they meet patient safety and clinical needs across their entire lifecycle.
- Good Software Engineering and Security Practices: Focuses on implementing robust software engineering, data quality assurance, and cybersecurity measures to ensure the reliability and integrity of AI/ML systems.
- Representative Data in Clinical Studies: Ensures that the data used to train and test AI/ML models accurately reflects the intended patient population, considering factors like age, gender, race, and other relevant characteristics to minimize bias.
- Independence of Training and Test Data: Requires that training and test datasets be kept independent to prevent biases and ensure that the AI/ML model is accurately evaluated and can generalize to real-world applications.
- Use of Best Available Reference Datasets: Encourages the use of the best available methods for developing reference datasets, ensuring that the data is clinically relevant and well-characterized to support robust AI/ML model development.
- Model Design Aligned with Intended Use: Stresses the importance of designing AI/ML models that are appropriate for the available data and the specific clinical use, while actively managing risks like overfitting and performance degradation.
- Focus on Human-AI Team Performance: Highlights the need to consider human factors in the design of AI/ML systems, ensuring that the interaction between humans and AI is optimized for the best overall performance in clinical settings.
- Testing Under Clinically Relevant Conditions: Emphasizes the importance of testing AI/ML devices in conditions that reflect their actual use, ensuring that they perform well in real-world clinical environments.
- Clear and Accessible Information for Users: Ensures that users, including healthcare providers and patients, are provided with clear, contextually relevant information about the AI/ML device, including its intended use, limitations, and performance.
- Monitoring and Re-training of Deployed Models: Involves continuous monitoring of AI/ML models after deployment to ensure ongoing performance and safety, with mechanisms in place to manage risks associated with re-training, such as overfitting or bias.
Summary of Predetermined Change Control Plans for Machine Learning-Enabled Medical Devices: Guiding Principles
The implementation of predetermined change control plans (PCCPs) helps manufacturers manage specific device modifications that would traditionally require regulatory approval before being marketed. PCCPs offer a strategic approach to:
- Synchronize regulatory procedures with the rapid and continuous nature of change management in machine learning-based medical devices (MLMDs).
- Mitigate risks efficiently and continuously through consistent monitoring, maintenance, and enhancements to device performance.
- Maintain stringent regulatory standards to ensure that the safety and effectiveness of the devices are not compromised.
The FDA, Health Canada, and MHRA collaboratively established five guiding principles for predetermined change control plans. These principles are rooted in the broader framework of Good Machine Learning Practice (GMLP), specifically reflecting the emphasis of principle 10, which highlights the importance of monitoring deployed models for performance and managing the risks associated with re-training.
Focused and Bounded:
A PCCP outlines specific modifications that a manufacturer plans to implement, limited to adjustments within the original intended use of the MLMD. This scope includes:
- The range and extent of planned modifications.
- Safety protocols for implementing these changes, including methods for validation and mechanisms to detect, halt, or reverse changes that do not meet specified performance criteria.
- The impact these planned changes will have on the device.
Risk-Based:
The reliability of a PCCP is strengthened when its design and execution are based on a risk-based approach that adheres to risk management principles. This approach is critical:
- Across the entire Total Product Lifecycle (TPLC), from initial design through deployment and usage.
- To ensure that individual and cumulative changes remain suitable over time for the device and its operational context.
Evidence-Based:
Evidence collected throughout the TPLC is essential to:
- Continuously ensure the device’s safety and effectiveness with a PCCP in place.
- Demonstrate that the benefits of the device outweigh its associated risks.
- Ensure that the risks are properly managed and controlled.
Key considerations include:
- The use of scientifically and clinically justified methods and metrics to measure device performance, aligned with the level of risk and consistent with other evidence gathered throughout the TPLC.
- Clearly defined methods for generating evidence that demonstrate the benefits and risks of the device before and after the implementation of changes outlined in the PCCP.
Transparent:
Transparency is essential for PCCPs, requiring clear and detailed communication with users and other stakeholders about the device’s performance and any modifications. This includes:
- A thorough description of the data used in development and modification processes, ensuring it accurately represents the intended user population.
- Comprehensive testing of planned changes.
- Detailed characterization of the device both before and after the implementation of changes.
- Ongoing monitoring and response mechanisms to detect and address any performance deviations.
Total Product Lifecycle (TPLC) Perspective:
Developing and implementing a PCCP from a TPLC perspective can:
- Enhance the quality and integrity of the PCCP by continually integrating the perspectives of all stakeholders and risk management practices throughout the TPLC.
- Align with existing regulatory, quality, and risk management systems to ensure device safety through proactive monitoring, reporting, and response to safety concerns.
Summary of Transparency for Machine Learning-Enabled Medical Devices: Guiding Principles
The FDA, Health Canada, and MHRA have outlined additional principles focused on ensuring transparency in machine learning-enabled medical devices (MLMDs). These principles are designed to expand on the foundation established by Good Machine Learning Practices (GMLP), with particular emphasis on:
- Principle 7: Emphasizing the performance of the collaborative human-AI team.
- Principle 9: Ensuring users are provided with clear, essential information.
Guiding Principles
The transparency of Machine Learning-Enabled Medical Devices (MLMDs) is guided by several key principles, focusing on various aspects such as audience, motivation, and methods of communication.
Who: Relevant Audiences
Transparency should be tailored to all relevant parties, including:
- Device Users: Healthcare professionals, patients, and caregivers who interact directly with the device.
- Healthcare Recipients: Patients who receive care through the use of these devices.
- Decision Makers: Support staff, administrators, payors, and governing bodies who influence device usage and patient outcomes.
Why: Motivation
Transparency is crucial for ensuring patient-centered care and maintaining device safety and effectiveness. It enables stakeholders to understand complex information, manage risks, identify errors, and uphold health equity by revealing how devices are developed and operate. Consistent transparency fosters trust, enhances fluency in using MLMDs, and encourages the adoption of beneficial technologies.
What: Relevant Information
The information shared should be appropriate to the MLMD in question, considering its benefits, risks, and the needs of its users. Key details include:
- Device Description: Medical purpose, function, intended users, and target populations.
- Workflow Integration: Explanation of inputs, outputs, and their impact on healthcare decisions.
- Performance and Risk Management: Information about device performance, risk management activities, and how the MLMD reaches its outputs.
- Product Development: Details on the underlying technology, training/testing data, and updates on the device's lifecycle, including limitations and known biases.
Where: Placement of Information
Information should be accessible through the device's user interface, encompassing all elements with which the user interacts. The software user interface should be optimized to respond to user needs, offering personalized and adaptive content via various modalities such as audio, video, on-screen text, and alerts.
When: Timing of Communication
Effective transparency involves timely communication throughout the device’s lifecycle. Detailed information is essential when acquiring, implementing, or modifying a device, and timely notifications should be provided for updates or new discoveries. Targeted communication, such as warnings or instructions, should be delivered at critical workflow stages or upon specific triggers.
How: Methods to Support Transparency
Supporting transparency requires a comprehensive understanding of users, environments, and workflows, achieved through human-centered design principles. This involves creating content that is accessible and usable, with an appropriate level of detail for the intended audience, and arranged to facilitate informed decision-making.
Summary of Predetermined Change Control Plans for Medical Devices: Draft Guidance for Industry and FDA Staff
Purpose of the Guidance
- Establishes a framework for manufacturers to develop Predetermined Change Control Plans (PCCPs) for medical devices.
- Aims to streamline regulatory approvals for modifications while maintaining device safety and effectiveness.
Definition of a Predetermined Change Control Plan (PCCP)
- A structured plan that outlines anticipated modifications to a medical device.
- Includes methodologies for implementing, verifying, and validating these changes.
- Enables manufacturers to make approved modifications without additional regulatory submissions.
- Description of anticipated changes – Details the modifications planned for the device.
- Rationale for changes – Justifies why the modifications are necessary or beneficial.
- Verification and validation methods – Specifies how the modifications will be tested and confirmed.
- Risk assessment – Evaluates the potential impact of modifications on device performance, safety, and effectiveness.
- Implementation strategy – Outlines the timeline and conditions under which changes will be made.
Regulatory Considerations
- The FDA expects PCCPs to be included in premarket submissions (e.g., 510(k), PMA, De Novo).
- Manufacturers must demonstrate that the planned changes will not compromise safety or effectiveness.
- The PCCP does not apply to all device modifications—only those that are pre-specified and validated within the plan.
Implementation and Compliance
- Manufacturers must document and track changes to ensure compliance with the approved PCCP.
- If changes exceed the scope of the PCCP, a new marketing submission may be required.
- The FDA retains oversight to ensure PCCPs align with existing regulatory requirements.
Benefits of PCCPs
- Faster implementation of safe and effective device modifications.
- Reduces regulatory burden by minimizing the need for repeated FDA submissions.
- Enhances innovation by allowing manufacturers to adapt to technological advancements efficiently.
Examples of Description of Modifications for PCCPs
This draft guidance offers an excellent look at different examples of modifications for PCCPs. It is important to note that the provided summaries of the modifications for PCCPs do not represent complete content expected in a Description of Modifications section in a PCCP.
Below are some of the examples of modifications for PCCPs the FDA provided in the Predetermined Change Control Plans for Medical Devices: Draft Guidance for Industry and Staff:
Example 1
This device is a microarray-based IVD cancer predisposition risk assessment system for over-the-counter use that is intended to detect five single nucleotide variants in BRCA1 and three single nucleotide variants in BRCA2 in saliva for the purposes of describing if a person is at increased risk of breast cancer, ovarian cancer, or prostate cancer.
Modifications that generally may be appropriate for inclusion in a PCCP:
- Addition of new single nucleotide variants
- Addition of insertion and deletion variants up to 20 base pairs
- Updates to the labeling that inform potential cross-reactive polymorphisms
Modifications that are generally not appropriate for inclusion in a PCCP:
- Addition of copy number variants
- Addition of a new gene
- Change in the collection device and sample type (e.g., saliva to buccal swab)
- Change from manual to automated process (e.g., for sample dilution)
Example 2
This device is an ion selective electrode IVD intended for use on a laboratory-based chemistry analyzer to quantify the concentrations of potassium ions in serum samples for the purposes of monitoring electrolyte balance in the diagnosis and treatment of diseases and conditions characterized by low or high blood potassium levels.
Modifications that generally may be appropriate for inclusion in a PCCP:
- Addition of lithium heparin plasma as a sample type
- Extension of sample stability claims (e.g., two hours at room temperature to four hours at room temperature)
- Addition of a new potassium ion selective electrode
Modifications that are generally not appropriate for inclusion in a PCCP:
- Addition of urine or capillary whole blood as a sample type
- Addition of at-home sample collection
- Addition of point-of-care use
Example 3
This device is a non-absorbable polyethylene surgical suture intended for soft tissue approximation or ligation.
Modifications that generally may be appropriate for inclusion in a PCCP:
- Change to a different non-novel sterilization method (e.g., Established Category A to Established Category B sterilization method)
- Extend shelf life using a different well-established method than what was provided for the initial shelf life testing
- Addition of sutures to the product line with different dimensions that are within the range of dimensions of those currently authorized
- Addition of dye with an appropriate FDA-listed color additive per 21 CFR Part 74 Subpart D
Modifications that are generally not appropriate for inclusion in a PCCP:
- Addition of antimicrobials
- Change in filament design to an atypical design (e.g., unique braiding patterns, anchors, or knots)
- Addition of a stiffening agent to the ends of the suture to address a recall
Example 4
This device is a multi-parameter physiological patient monitor with arrhythmia detection and alarms for use in a hospital environment.
Modifications that generally may be appropriate for inclusion in a PCCP:
- Hardware and software updates to introduce compatibility with a newly cleared monitoring parameter/module
- Change to a new wireless card that has different technical specifications than those for the authorized device
- Change to the software to upgrade to a new version of the currently supported operating system (OS) on the basis of a risk assessment that shows changes between major OS versions could significantly impact the software’s performance.
- Change to the visualization of patient monitoring data (e.g., heart rate) in the user interface.
- Addition of new parameter trend graphs for use in active patient monitoring.
Modifications that are generally not appropriate for inclusion in a PCCP:
- Addition of a new physiological parameter to be monitored (e.g., blood oxygen level, temperature).
- Addition of a novel physiological or predictive index or algorithm (e.g., new algorithm to predict risk of patient deterioration).
- Significant changes to the alarm architecture.
- Significant changes to the design of the printed circuit board.
Summary of Marketing Submission Recommendations for a Predetermined Change Control Plan for Artificial Intelligence-Enabled Device Software Functions
Purpose of the Guidance
- Provides recommendations for including a Predetermined Change Control Plan (PCCP) in marketing submissions for devices featuring Artificial Intelligence-Enabled Device Software Functions (AI-DSFs).
- Aims to support iterative improvements in AI-DSFs while ensuring device safety and effectiveness.
Definition of Key Terms
- Artificial Intelligence (AI): A machine-based system that can, for a given set of human-defined objectives, make predictions, recommendations, or decisions influencing real or virtual environments.
- Machine Learning (ML): A set of techniques that can be used to train AI algorithms to improve performance at a task based on data.
- Artificial Intelligence-Enabled Device Software Function (AI-DSF): A device software function that implements an AI model.
- Predetermined Change Control Plan (PCCP): Documentation describing planned modifications to an AI-DSF, including:
- Description of Modifications
- Modification Protocol
- Impact Assessment
Scope of the Guidance
- Applies to AI-DSFs that manufacturers intend to modify over time.
- Relevant to devices reviewed through 510(k), De Novo, and PMA pathways.
- Includes the device constituent part of device-led combination products when the device constituent part includes an AI-DSF.
Components of a PCCP
- Description of Modifications: Detailed explanation of each planned change to the AI-DSF.
- Modification Protocol: Methodology for developing, validating, and implementing the planned modifications.
- Impact Assessment: Evaluation of how the modifications may affect the device's safety and effectiveness.
Regulatory Considerations
- Including a PCCP in a marketing submission allows manufacturers to implement specified modifications without submitting new marketing applications for each change.
- The PCCP should outline modifications that would typically require a new marketing submission if not pre-approved.
- Manufacturers must ensure that modifications are implemented in accordance with the authorized PCCP to maintain device safety and effectiveness.
Implementation and Compliance
- Manufacturers are responsible for documenting and tracking changes made under an authorized PCCP.
- If modifications exceed the scope of the authorized PCCP, a new marketing submission may be necessary.
- The FDA retains oversight to ensure that PCCPs align with regulatory requirements and that devices remain safe and effective throughout their lifecycle.
Benefits of Implementing a PCCP
- Facilitates timely and efficient updates to AI-DSFs, promoting innovation.
- Reduces the regulatory burden by minimizing the need for multiple marketing submissions.
- Ensures that iterative improvements maintain the safety and effectiveness of AI-enabled medical devices.
Example AI-DSF Scenarios Employing PCCPs
Below is a list of examples directly from this guidance. Due to the complexity of AI-DSFs, providing a comprehensive description of all relevant considerations for a complete PCCP is not practical for the limited examples presented below.
Example 1: Patient Monitoring Software
Background:
The device is an AI-DSF intended for use in high-acuity healthcare environments (e.g., an intensive care unit). The software obtains physiological signals (e.g., electrocardiogram, blood pressure, pulse oximetry) from a primary patient monitor. The physiological signals are processed and analyzed by an AI model to detect patterns that occur at the onset of physiologic instability. When physiologic instability is detected, an audible alarm signal is generated to indicate that prompt clinical action is needed to prevent potential harm to the patient. The AI-enabled medical device was authorized with a PCCP.
Brief Overview of Pre-Specified Modification:
The manufacturer would like to re-train the AI model with more data to reduce the false alarm rate while maintaining or increasing sensitivity to the onset of physiologic instability. The baseline sensitivity is y%. The manufacturer would like to demonstrate a significant improvement in the false-alarm rate while the sensitivity remains within a pre-specified non-inferiority margin of z% when compared with the original device, i.e., the version of the device without any modifications.
Post-Authorization Modification Scenarios:
Modification Scenario 1: Modification related to quantitative measures of device performance, as specified in the PCCP and implemented in accordance with the PCCP
In accordance with the Modification Protocol, data were collected and used to re-train the AI model. The modified AI model was tested per the methods specified in the Modification Protocol. The results demonstrated that the false alarm rate was significantly reduced while the mean sensitivity estimate was statistically within the proposed non-inferiority margin of the baseline sensitivity y%. Labeling was updated in accordance with the modified AI-DSF performance, and communication was provided to the device users. Because the device modification was specified in the PCCP, and it was implemented in conformance with the PCCP, the device modification would not require a new marketing submission. The manufacturer should document the modification that was specified in the PCCP in accordance with their quality system.
Modification Scenario 2: Modification beyond quantitative measures of device performance, which was not specified in the PCCP
In accordance with the Modification Protocol, the manufacturer re-trained their AI model using additional data to improve the sensitivity. Analytical validation demonstrated that the revised AI model has the same false alarm rate and sensitivity as the previous version. However, the manufacturer also noticed that the modified AI model maintained the same sensitivity and can now also predict physiologic instability in advance of its onset, which the previous version of the AI model could not do. The manufacturer would like to update the device’s indications for use to reflect this additional performance claim to predict physiologic instability in advance of its onset, which was not previously included in the PCCP. The methods used for analysis, performance, and statistics were not specified in the PCCP for predicting a future state. Because this modification that was not included in the PCCP could significantly affect the safety or effectiveness of the device, a new marketing submission would be required.
Example 2: Skin Lesion Software
Background:
The device is an AI-DSF that analyzes images of skin lesions by identifying and characterizing its features (e.g., color, quantification of area change over time) to aid in diagnosis. It was validated with a specific camera and is intended to be used by a primary healthcare provider. The AI-enabled medical device was authorized with a PCCP.
Brief Overview of Pre-Specified Modification:
The manufacturer would like to extend the AI-DSF for use on additional general-purpose computing platforms, including smartphones and tablets. The general-purpose computing platform must include a two-dimensional camera that meets the minimum specifications defined in the PCCP. The updated device must achieve a minimum performance defined in the Modification Protocol using a specified methodology.
Post-Authorization Modification Scenarios:
Modification Scenario 1: Modification in input, as specified in the PCCP and implemented in accordance with the PCCP
The manufacturer’s analytical validation demonstrated that the AI-DSF can be deployed on two additional smartphones that have image acquisition specifications that meet the minimum specifications provided in the PCCP. The analytical performance using the new image acquisition systems was found to be statistically equivalent to the baseline performance, as specified in the Modification Protocol. Labeling was updated to reflect the new AI-DSF compatibility with the additional smartphones, which may increase access of the AI-DSF in the healthcare community. Communication updates on device compatibility were also provided. Because the device modification was specified in the PCCP, and it was implemented in conformance with the PCCP, the device modification would not require a new marketing submission. The manufacturer should document the modification that was specified in the PCCP in accordance with their quality system.
Modification Scenario 2: Modification in input, which was not specified in the PCCP
The manufacturer would like to deploy a modified AI model that uses images captured by a thermographic camera; however, the new camera technology was not specified in the PCCP. Because this modification that was not included in the PCCP could significantly affect the safety or effectiveness of the device, a new marketing submission would be required.
Modification Scenario 3: Modification related to the device’s use and performance, which was not specified in the PCCP
The manufacturer would like to distribute a new version of the AI-DSF that is patient-facing. The AI-DSF would provide an analysis of the physiological characteristics of skin lesions, as it does currently, and direct patients to follow up with a dermatologist based on the preliminary analysis of the malignancy of the skin lesion. The modification introduces many new, unconsidered risks that were not yet mitigated in the current PCCP, given that the modified AI-DSF will be patient-facing. Because this modification that was not included in the PCCP could significantly affect the safety or effectiveness of the device, a new marketing submission would be required."
Artificial Intelligence-Enabled Device Software Functions: Lifecycle Management and Marketing Submission Recommendations
Purpose of the Guidance
- Provides recommendations for marketing submissions of devices incorporating Artificial Intelligence-Enabled Device Software Functions (AI-DSFs).
- Emphasizes a Total Product Life Cycle (TPLC) approach to ensure the safety and effectiveness of AI-DSFs.
Scope
- Applies to devices with AI-DSFs reviewed through 510(k), De Novo, and PMA pathways.
- Includes device constituent parts of combination products containing AI-DSFs.
Total Product Life Cycle (TPLC) Approach: General Principles
- Encourages continuous monitoring and maintenance of AI-DSFs throughout their lifecycle.
- Advocates for proactive management of risks associated with AI-DSFs.
Marketing Submission Content Recommendations
- Device Description: Comprehensive details of the AI-DSF, including its architecture, algorithms, and data sources.
- User Interface and Labeling: Clear instructions and information for users to ensure proper interaction with the AI-DSF.
- Risk Assessment: Identification and evaluation of potential risks associated with the AI-DSF, including those related to algorithm performance and data integrity.
- Data Management: Strategies for handling data used by the AI-DSF, ensuring its quality, integrity, and security.
- Model Description and Development: Information about the model and device design, including its biases and limitations, helps the FDA assess the safety and effectiveness of the AI-enabled device and determine its performance testing specifications.
- Validation: For an AI-enabled device, validation ensures that the device, as used by users, performs its intended function safely and effectively while consistently meeting its relevant performance specifications.
- Device Performance Monitoring: Manufacturers of AI-enabled devices should proactively monitor, identify, and address performance changes, as well as variations in device inputs and usage context that could impact device performance.
- Cybersecurity: For AI-enabled devices classified as “cyber devices” under section 524B(c) of the FD&C Act, the recommendations in this guidance aim to assist manufacturers in fulfilling their obligations under section 524B of the FD&C Act.
- Public Submission Summary: Transparency is essential for premarket authorization and patient care, particularly for AI-enabled devices, which rely on data-driven algorithms that may exhibit a degree of opacity.
Additional Key Concepts Throughout the Recommendations
Quality System Documentation
- Documentation of processes and procedures ensuring the AI-DSF's quality throughout its lifecycle.
- Includes design controls, software validation, and corrective and preventive actions.
User Interface and Labeling
- User Interface: Design considerations ensuring usability and minimizing user errors.
- Labeling: Comprehensive information for users, including indications for use, contraindications, warnings, and instructions.
Performance Testing
- Validation of the AI-DSF's performance under expected conditions of use.
- Verification that the AI-DSF meets predefined specifications and performance criteria.
How Ketryx Can Help with FDA AI Guidance
Ketryx helps companies release software faster and in accordance with their PCCP by enabling developers to utilize modern development tools and practices while equipping quality teams with automated documentation and testing. Ketryx also automatically updates traceability and enables users to create engineering controls to ensure they are following their PCCP. Our software empowers manufacturers to use the tools that are used by software professionals today to develop and update software, like Jira and GitHub.
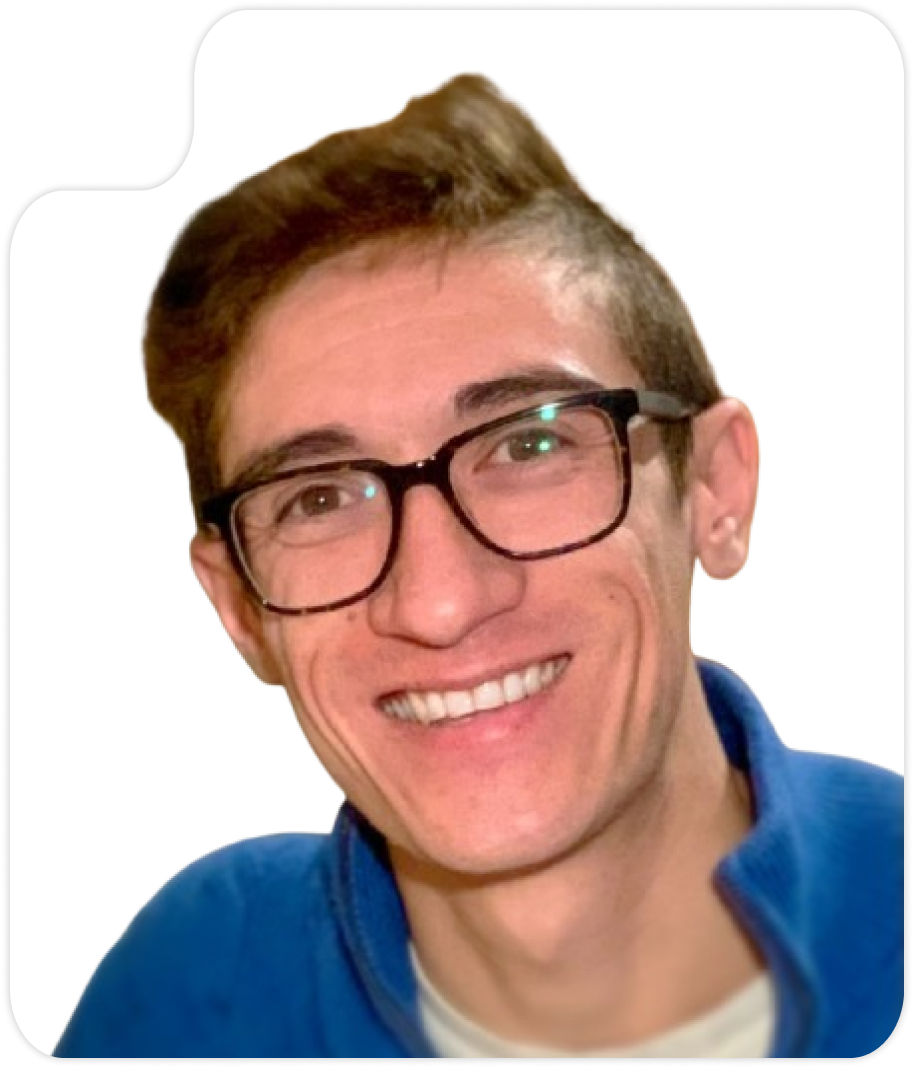
Lee Chickering is a Client Operations Manager at Ketryx and an expert in quality assurance and regulatory compliance, specializing in bridging quality management and customer success to drive operational excellence in the life sciences industry. With a diverse background spanning manufacturing, project management, and compliance at companies like Amgen, he has led the implementation of Quality Management Systems (QMS) aligned with ISO 13485, ISO 14971, and IEC 62304. Passionate about advancing quality in life sciences, he thrives on collaborating with organizations to enhance efficiency, compliance, and innovation.