Navigating the FDA's New Draft Guidance on AI-Enabled Devices
Table of Contents
The FDA's new draft guidance on AI-enabled devices offers a framework for medical device manufacturers seeking to pursue AI/ML innovation while staying compliant. The draft guidance provides firm direction on how to apply a TPLC approach to the design, development, and ongoing maintenance of safe AI-powered devices. The draft guidance document is a must-read for any developer or QA/RA specialist who plans to submit an AI device in 2025.
Please note that though the recommendations in the guidance are non-binding, they do represent the FDA’s latest thinking on the subject and may soon become final. We will be watching to see how this guidance evolves over time.
Why This Guidance Matters
While the FDA has already approved more than 1,000 AI-enabled devices to date, the lack of precedence for AI submissions made it challenging for regulatory professionals to know what exactly they needed to submit to the FDA when seeking device approval. This new guidance provides much-needed clarity, especially for developers and data scientists working on AI models in healthcare.
A Focus on Transparency
The guidance places a significant emphasis on model transparency for AI-enabled devices, since many models can feel like a “black box” to users who don’t know how the model was built. The document reflects the desire from both patients and clinicians to see manufacturers provide more information about when and how AI is used in devices. The FDA recognizes that patients and clinicians are hesitant to use AI devices without an understanding of how they were developed, hence they are requiring that labelling provided by the manufacturers would empower end users with model information. The guidance encourages the use of “model cards,” short documents that include key information about an AI model, such as how the model functions, what data it was trained on, and how performance was validated.
Minimizing Bias in Data
The new draft guidance addresses the importance of mitigating AI bias, which the FDA defines as the potential tendency to produce incorrect results that affect the safety and effectiveness of the device in the intended use population. The document indicates that the FDA may soon expect companies to use training data for AI model development that sufficiently represents the intended use population, including age, race, ethnicity, and sex considerations. Monitoring and validation strategies should account for the risk of bias by including diverse datasets and subgroup analyses. The draft guidance also discusses the importance of proper data management, including data cleaning, annotation, and validation, to identify and reduce bias. The agency wrote that manufacturers should provide information in their submissions about how data were collected, the size and limitations of each dataset, a description of outreach approaches utilized to encourage dataset diversity, and how they are making sure that results can be used across a variety of populations and clinical settings.
Quality Management and Risk Controls
The guidance emphasizes that maintaining a quality management system (QMS) that includes AI from the early stages of model design and development is crucial. Organizations that have not documented data origin and decision-making processes for their AI/ML-enabled devices will struggle to do this work retroactively.
A critical aspect of the guidance involves integrating quality system documentation into submissions. The FDA clarifies that quality system regulation (QSR) documentation, such as performance monitoring and risk management, can be submitted as evidence of safety and effectiveness. This allows for ongoing assurance of device safety through the QMS rather than solely within the design history file (DHF).
Additionally, the standard that Ketryx's founder, Erez Kaminski, helped write—AAMI TIR34971 Application of ISO 14971 to machine learning in artificial intelligence—is referenced via CR34971 in the risk management section of the draft guidance.
Labeling and User Interface Considerations
The guidance introduces a key shift by defining the user interface as a component of device labeling. This means that on-screen warnings, tooltips, and visual indicators will be subject to the same standards as traditional labeling materials. Including these elements directly within the device helps ensure critical safety information is always visible to the end user.
Addressing AI-Specific Cybersecurity Risks
A notable addition to the guidance is its focus on cybersecurity risks specific to AI models. The FDA highlights concerns such as data poisoning and model tampering, urging manufacturers to integrate cybersecurity controls directly into risk management processes. Cyber threats could manipulate training data to introduce or exacerbate bias, use adversarial examples to exploit existing biases, or embed backdoors that trigger biased behavior. Controls against such risks include data validation, anomaly detection, and differential privacy techniques. As cybersecurity is an adversarial space, expect AI-specific risks to become more prevalent over time.
Postmarket Surveillance and Continuous Improvement
The FDA encourages manufacturers to adopt proactive postmarket surveillance strategies for AI-enabled devices, including a postmarket performance monitoring plan to maintain performance over time. This involves embedding performance monitoring directly into the device software, ensuring that ongoing safety and effectiveness can be tracked throughout the product lifecycle. Those manufacturers who decide to include a postmarket monitoring plan in their premarket submissions should include the methods they plan to use to assess changes in model performance and how they are going to deploy updates or corrective actions in the case of performance changes.
The Connection to PCCP Guidance
The new draft guidance arrived on the heels of final guidance from the FDA on the Predetermined Change Control Plan (PCCP) framework. While the PCCP outlines how to manage changes to AI models post-clearance, this new guidance clarifies the submission requirements for initial market entry. Together, they offer a comprehensive pathway for managing both initial compliance and iterative updates to AI-enabled devices. In the new draft guidance, the FDA encouraged manufacturers to consider a PCCP so that they can make changes to continuously improve model performance without having to resubmit for each change.
Validation for AI-Enabled Devices
The draft guidance emphasizes two relevant forms of validation for AI-enabled medical devices: performance validation and human factors validation (or an evaluation of usability). Performance validation is meant to confirm that the device meets its intended use and that performance requirements are consistently met. Human factors validation and an evaluation of usability address whether all intended users can achieve specific goals while using the device and should confirm that users can consistently interact with the device safely and effectively. Performance validation may use a variety of testing methods to measure the statistical performance of the model under testing conditions, and human factors validation testing is focused on understanding how various users effectively use a device in context.
Notably, the FDA highlights that a holistic approach to validation testing could include both standalone performance evaluation of the model and a human-device team performance evaluation. Depending on the device, focus could be placed more on one or the other.
Together, these two types of validation give the FDA information on how the device may be used and perform in real-world situations. This comprehensive approach helps mitigate risks associated with human error, cognitive overload, or misinterpretation of AI outputs, ultimately supporting safer adoption of AI in healthcare.
Key Takeaways for MedTech Companies
This new draft guidance represents a positive step for the MedTech industry, offering clarity on how to document, validate, and monitor AI-enabled devices effectively. To stay ahead, manufacturers should:
- Implement a robust QMS early in the development process, including policies and processes related to AI in their devices.
- Maintain detailed documentation of data management and model performance.
- Integrate user interface design with labeling requirements.
- Address AI-specific risks, including cybersecurity threats.
- Plan for proactive postmarket surveillance and model versioning.
By aligning with these guidelines, MedTech companies can streamline regulatory submissions while ensuring patient safety and device effectiveness.
The FDA's evolving approach to AI regulation reflects the need for both technical clarity and regulatory adaptability, ultimately benefiting both manufacturers and patients through safer, more transparent AI-enabled devices.
How to Build Compliant AI-Enabled Devices
Ketryx helps you build your AI-powered medical device in a way that is compliant with FDA guidance by simplifying change management, ensuring traceability, and accelerating development across your AI lifecycle. Ketryx allows MedTech teams to validate, document, and manage their AI/ML models, with support for PCCPs and various AI governance practices. Automate traceability between AI requirements in Jira and tests in Git, enforce your QMS throughout your systems, and continuously monitor model drift to keep your device safe and effective. Empower your development teams to use their preferred tools while maintaining compliance with FDA regulations so you can accelerate AI innovation without sacrificing quality and safety.
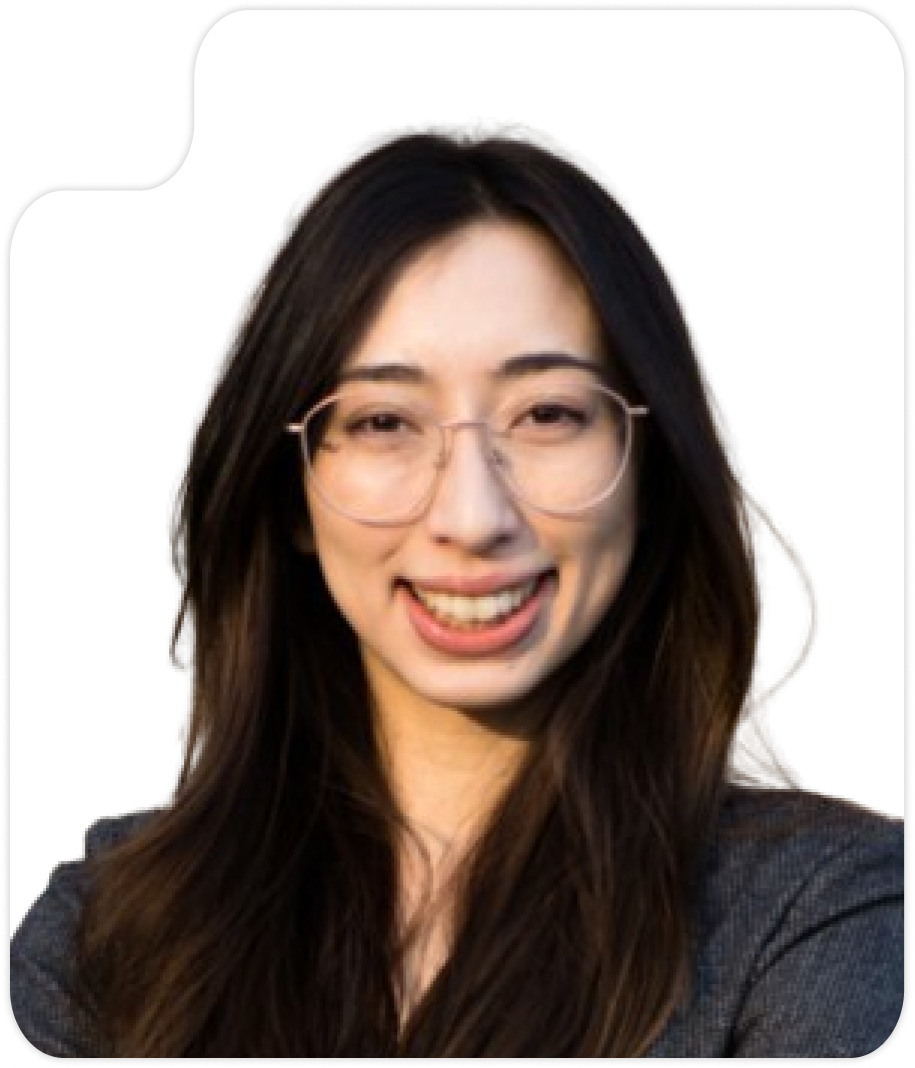
Jenn Dixon leads quality and regulatory client engagements at Ketryx, where she provides coaching and support for clients wishing to adopt the best Software Medical Device practices. Jenn brings extensive expertise in quality assurance, regulatory affairs, and project management from previous roles in the MedTech industry with a proven ability to develop and apply best practices that optimize both teams and processes.
Prior to Ketryx, Jenn was Director of QA/RA at Omniscient Neurotechnology, overseeing global regulatory submissions, maintaining ISO 13485 compliance, and managing cross-functional teams in areas such as post-market surveillance and Good Machine Learning Practices (GMLP). Jenn also served as a Design Assurance Specialist and Technical Project Manager, where she excelled in risk management, audit readiness, and leading agile development teams to deliver innovative solutions.
Jenn began her career at Synaptive Medical, supporting ISO 13485 compliance transitions and leading UDI implementation. She holds a Bachelor’s degree in Engineering from the University of Toronto.